

The resulting algorithm is formally similar, except that every dot product is replaced by a nonlinear kernel function. However, in 1992, Bernhard Boser, Isabelle Guyon and Vladimir Vapnik suggested a way to create nonlinear classifiers by applying the kernel trick (originally proposed by Aizerman et al. coordonnes de manire que lhyperplan tangent en x X ait pour quation T. The original maximum-margin hyperplane algorithm proposed by Vapnik in 1963 constructed a linear classifier. vectoriel sous-jacent A, et G le k-groupe Autk(Y ) cest videmment un. For example, if a space is 3-dimensional then its hyperplanes are the 2-dimensional planes, while if the space is. In geometry, a hyperplane is a subspace whose dimension is one less than that of its ambient space. A plane is a hyperplane of dimension 2, when embedded in a space of dimension 3. In the case of support-vector machines, a data point is viewed as a p either Two intersecting planes in three-dimensional space. Suppose some given data points each belong to one of two classes, and the goal is to decide which class a new data point will be in. H 3 separates them with the maximal margin.Ĭlassifying data is a common task in machine learning. The support-vector clustering algorithm, created by Hava Siegelmann and Vladimir Vapnik, applies the statistics of support vectors, developed in the support vector machines algorithm, to categorize unlabeled data. suggr une technique de quantification vectorielle dans laquelle la comparaison est.
HYPERPLAN VECTORIEL DOWNLOAD
When data are unlabelled, supervised learning is not possible, and an unsupervised learning approach is required, which attempts to find natural clustering of the data to groups, and then map new data to these formed groups. Download scientific diagram 6-Schma dhyperplan pour des donnes.

In addition to performing linear classification, SVMs can efficiently perform a non-linear classification using what is called the kernel trick, implicitly mapping their inputs into high-dimensional feature spaces.
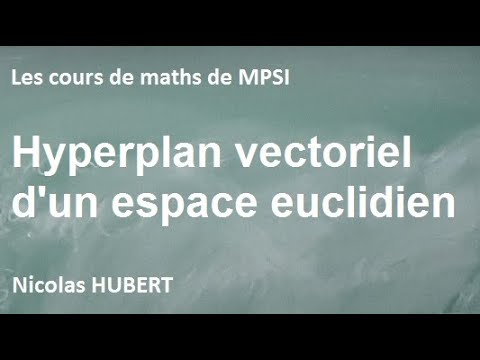
New examples are then mapped into that same space and predicted to belong to a category based on which side of the gap they fall. SVM maps training examples to points in space so as to maximise the width of the gap between the two categories. Given a set of training examples, each marked as belonging to one of two categories, an SVM training algorithm builds a model that assigns new examples to one category or the other, making it a non- probabilistic binary linear classifier (although methods such as Platt scaling exist to use SVM in a probabilistic classification setting). Developed at AT&T Bell Laboratories by Vladimir Vapnik with colleagues (Boser et al., 1992, Guyon et al., 1993, Cortes and Vapnik, 1995, Vapnik et al., 1997 ) SVMs are one of the most robust prediction methods, being based on statistical learning frameworks or VC theory proposed by Vapnik (1982, 1995) and Chervonenkis (1974). In machine learning, support-vector machines ( SVMs, also support-vector networks ) are supervised learning models with associated learning algorithms that analyze data for classification and regression analysis.
